In the realm of horse racing, where intuition and experience often take the lead, a revolutionary tool is changing the game: EstoTurf. Combining sophisticated data science techniques with deep domain expertise, EstoTurf offers a powerful approach to predicting race outcomes. This article delves into the intricacies of EstoTurf, examining the data science methods that underpin its predictive prowess.
The Genesis of EstoTurf
EstoTurf emerged from the need to bring a data-driven edge to horse racing predictions. Traditional methods often relied on historical performance, jockey and trainer statistics, and other qualitative factors. EstoTurf, however, integrates a more comprehensive and nuanced approach by harnessing advanced data science techniques. Its goal is to provide more accurate and reliable predictions, reducing the inherent unpredictability of horse racing.
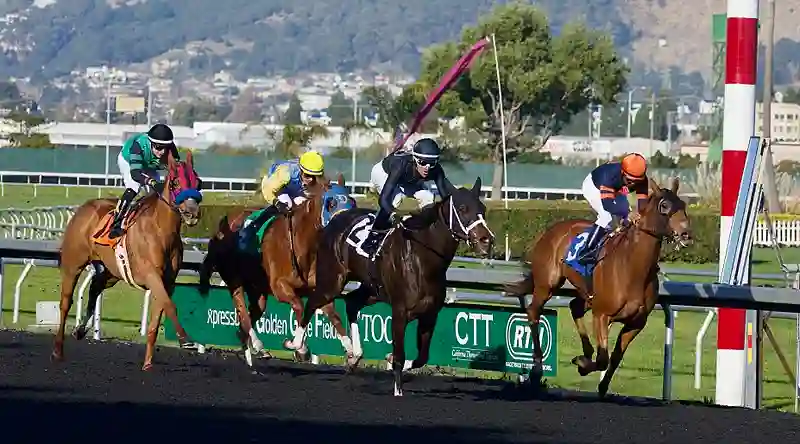
Data Collection and Preprocessing
At the heart of EstoTurf’s predictive model is a massive amount of data. The first step in this process is data collection. EstoTurf gathers a wide array of data points, including:
- Horse Performance History: Past race results, speed figures, and performance on different tracks.
- Jockey and Trainer Statistics: Historical performance metrics and win rates.
- Track Conditions: Weather data, track surface type, and condition.
- Horse Health and Training Data: Veterinary records, training logs, and recovery times.
Once collected, this data undergoes rigorous preprocessing. This step involves cleaning the data to handle missing values, outliers, and inconsistencies. Standardization and normalization techniques are applied to ensure that the data is in a usable format, which is crucial for building a robust predictive model.
Feature Engineering
Feature engineering is a critical component of EstoTurf’s data science approach. It involves creating new features from the existing raw data to enhance the model’s predictive power. For instance:
- Speed Adjustments: Adjusting a horse’s speed figures based on track conditions and race distance.
- Form Indicators: Developing metrics that capture a horse’s recent performance trends.
- Jockey and Trainer Efficiency: Creating features that quantify the effectiveness of jockeys and trainers based on their past performance.
These engineered features help in capturing complex relationships within the data that raw metrics might miss.
Predictive Modeling
With preprocessed data and engineered features in hand, EstoTurf employs various predictive modeling techniques to forecast race outcomes. Key methods include:
1. Regression Analysis
Regression models, such as linear and logistic regression, are used to predict continuous outcomes (e.g., finishing times) or categorical outcomes (e.g., winning probability). These models help identify relationships between dependent variables (e.g., race outcomes) and independent variables (e.g., horse performance metrics).
2. Machine Learning Algorithms
EstoTurf leverages advanced machine learning algorithms, including:
- Random Forests: An ensemble method that combines multiple decision trees to improve prediction accuracy and handle complex data relationships.
- Gradient Boosting Machines (GBM): An algorithm that builds models sequentially to correct the errors of previous models, enhancing predictive performance.
- Neural Networks: Deep learning models that can capture intricate patterns in large datasets, offering high accuracy for complex predictions.
3. Ensemble Methods
Combining multiple models, known as ensemble methods, helps in improving prediction robustness. EstoTurf utilizes techniques like stacking, where predictions from various models are aggregated to make the final prediction. This approach minimizes individual model biases and enhances overall accuracy.
Evaluation and Validation
Evaluating and validating predictive models is crucial to ensure their reliability. EstoTurf employs several metrics to assess model performance, including:
- Accuracy: The proportion of correct predictions made by the model.
- Precision and Recall: Metrics that measure the model’s performance in identifying winners versus non-winners.
- Cross-Validation: A technique where the data is divided into multiple subsets to test the model on different parts of the dataset, ensuring that the model generalizes well to new data.
Regular backtesting against historical race data helps in fine-tuning models and adjusting for any shifts in racing trends or conditions.
Real-World Application and Impact
The practical application of EstoTurf’s predictions extends beyond theoretical accuracy. By providing actionable insights and predictions, EstoTurf offers several benefits:
- Informed Betting: Bettors can make more informed decisions based on data-driven predictions rather than relying solely on intuition or conventional wisdom.
- Improved Performance Analysis: Trainers and jockeys can gain insights into performance trends and optimize their strategies accordingly.
- Enhanced Fan Engagement: Accurate predictions and analyses can increase interest and engagement in the sport, offering fans deeper insights into races.
Conclusion
EstoTurf represents a significant leap forward in the field of horse racing predictions. By integrating advanced data science techniques with extensive racing data, it provides a sophisticated tool for enhancing prediction accuracy. The combination of thorough data preprocessing, feature engineering, and sophisticated modeling techniques ensures that EstoTurf remains at the forefront of predictive analytics in horse racing. As the technology continues to evolve, EstoTurf stands as a testament to the power of data science in transforming traditional sports betting and racing strategies.